DeepTHM
Deep learning-based Tool Health Management
Deep Learning-based Tool Health Management (DeepTHM) is a real-time monitoring solution that can accurately determine the wear and broken status of tools in operation.
The current state of a tool is monitored by dividing it into four stages: normal, alert, alarm, and broken. These four stages are determined by the level at which a tool begins to be used and wears out. The alarm level means poor machining quality and a high probability of breakage and can be interpreted as an immediate tool change signal. The broken stage means severe wear or physical deformation and requires immediate discontinuance. In this way, reliable predictive maintenance is possible through real-time identification of all tools of a machine tool. DeepTHM can also generate a emergency signal to stop a machine tool when tool breakage is detected.
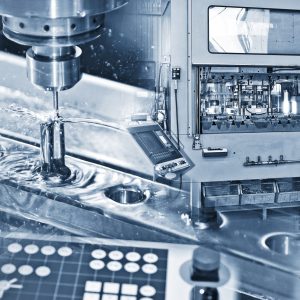
Real-Time Monitoring Solution Without Stop Machinging
In order to determine the condition of tools, the machine can be stopped during machining and then the tool wear value can be measured directly. However this approach can cause productivity decreasing problems. Performing preventive maintenance on tools to ensure their health status is one way, but this is also not an efficient way to solve that kind of problems. Therefore, the system should be able to monitor the current state of tools in real time and respond immediately when a problem occurs. It is a realistic alternative to indirectly estimate the tool wear state by analyzing the data collected using multiple sensors.
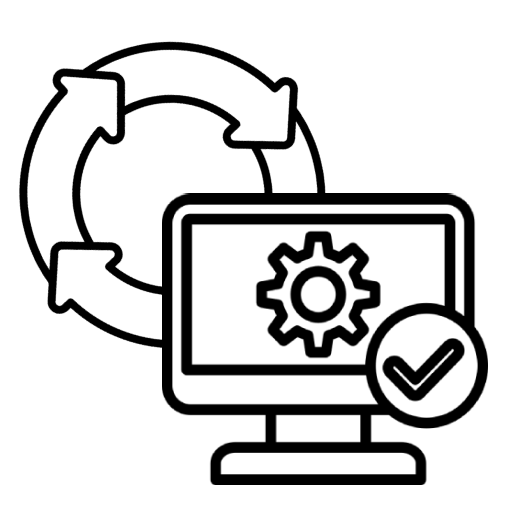
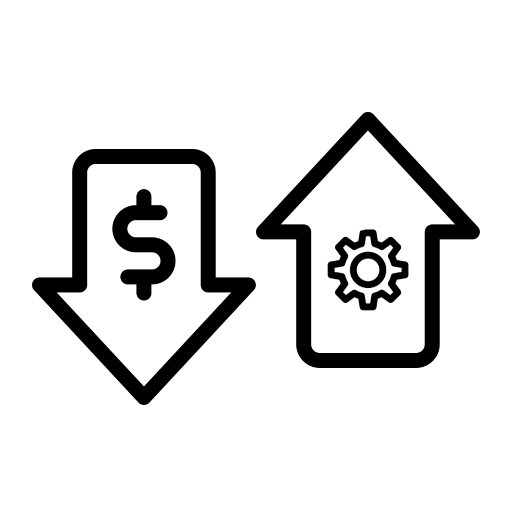
Reduce Basic Maintenance Cost, Improve of Factory Productivity
Accurate prediction of tool wear conditions enables timely, predictive maintenance. Through this, it is possible to reduce basic maintenance cost by minimizing the occurrence of unplanned failures. In addition, it contributes to the improvement of factory productivity by maximizing the operation efficiency of machine tools. Ultimately, by maintaining the best tool condition, the quality of workpiece is improved and the production defect rate is reduced.
Applicable to All Kinds of Tools Driven by a Spindle Motor
DeepTHM is applicable to all kinds of tools driven by a spindle motor. The followings are representative tools that can predict tool wear: Bit tool, drill, reamer, tap, milling cutter, hob, grinding wheel, and broach.
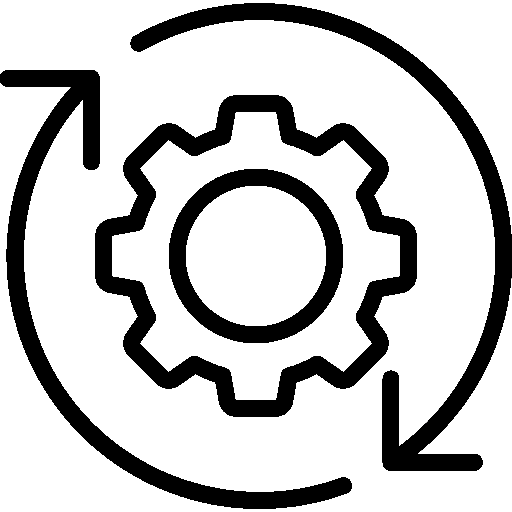
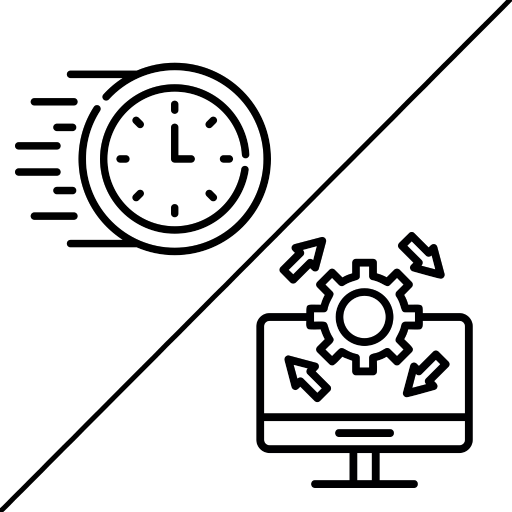
Fast Initial Installation, Continuously Improve Performance by Further Training the Model
DeepTHM is implemented based on anomaly detection machine learning and deep learning technology, so it has the advantage of fast initial installation. It is also possible to continuously improve performance by further training the model using data collected during operation.
Use Case – MCT Processing Process
The MCT machine is a key machine in the modern industry that can automatically replace tools and work in sophisticated bulk such as drilling and milling. The MCT, which operates 24 hours a day without a break, may break the tool unexpectedly. Failure to detect breakage of these tools can result in huge amounts of defective products.
Previously, the condition of the tool was identified by inspecting it for a certain period of time or by inspecting the person of the finished product. The problem with this method is that after producing a large amount of defective products, it is inevitable to detect damage to the tool.
The input current of the spindle motor of the MCT implements a deep learning model that detects wear and tear of the tool, allowing for pre-prediction before breakage and immediate detection of breakage to eliminate additional losses.
This solution reduces the number of unidentified tool breaks by 75%. In addition, the amount of loss due to the disposal of processed products has decreased by 11%.