DeepMV
Deep learning-based Machine Vision
Deep learning-based Machine Vision (DeepMV) is a machine vision solution based on artificial intelligence and deep learning technology to detect and classify various product defects on a manufacturing site.
DeepMV automatically detects the presence and location of defects from product images taken with area scan, line scan, or hyperspectral cameras, etc. In addition, it is also a basic function to additionally determine the type and attribute information of the detected defect. It is also possible to transmit information such as the location and classification of defects to an external system, by a specific integration work. DeepMV recommends the optimal and economical GPU system by comprehensively considering the size, speed, and performance of defect detection.
DeepMV enables consistent quality control regardless of the skill level of an inspector. Specifically, it can automatically aggregate data such as type, location, size, and number of defects related to all defects in production. Statistical analysis of the recorded defect data also makes it possible to identify the cause of the defect and diagnose production process vulnerabilities. Machine vision solutions can be used not only for defect detection, but also for mark, text, barcode recognition and precision measurement, and thus can be used as a fundamental tool for building a smart factory.
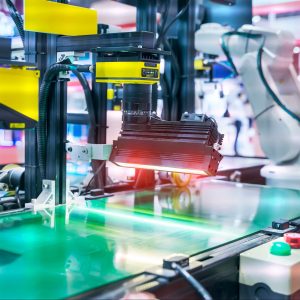
One-Class Anomaly Detection Model That Trains a Deep Learning Model Using Only Normal Data
Usually, it takes a lot of data to build a good deep learning model, but it is quite difficult to obtain defect data. Under this structure, it is difficult to expect the best detection performance unless sufficient data can be obtained. DeepMV uses a one-class anomaly detection model that trains a deep learning model using only normal data, not defect data.
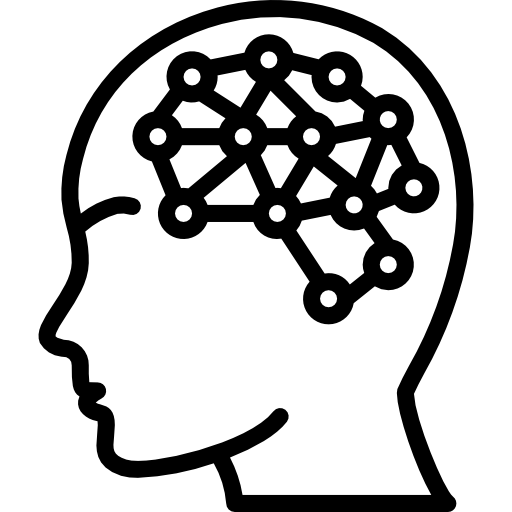
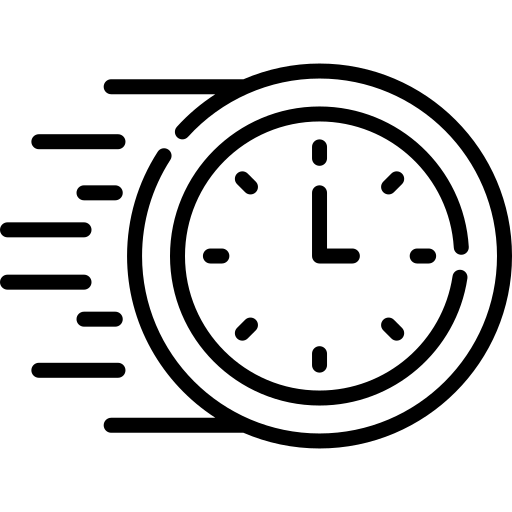
Enabling Rapid Initial Deployment
DeepMV is implemented based on anomaly detection deep learning technology, enabling rapid initial deployment. Taking hundreds of normal images is sufficient to build an immediate defect detection model. DeepMV does not require a reprogramming process for the detection algorithm during installation, so the initial installation process is quick and immediate field application is possible.
High Recall and Precision
Before the present AI technology was used, the method of artificially finding the features of a defect and applying it based on rules written by people was the mainstream. Machine vision technology based on deep learning is robust against changes in defect location, size, shooting angle, and lighting conditions. In addition, it minimizes false detection by satisfying high recall and precision at the same time. Depending on the type of product, defects of various sizes can be detected from 10 µm units to 10 m units.
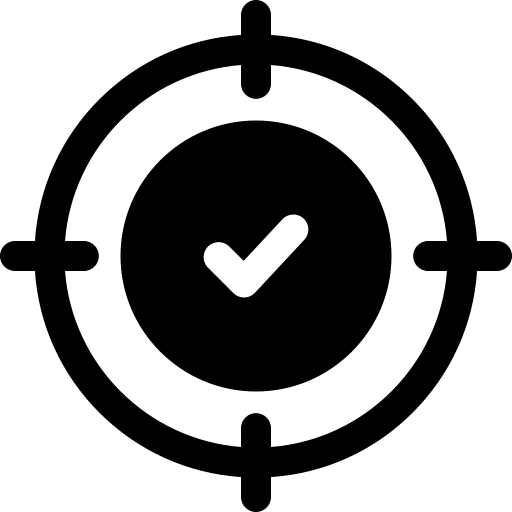
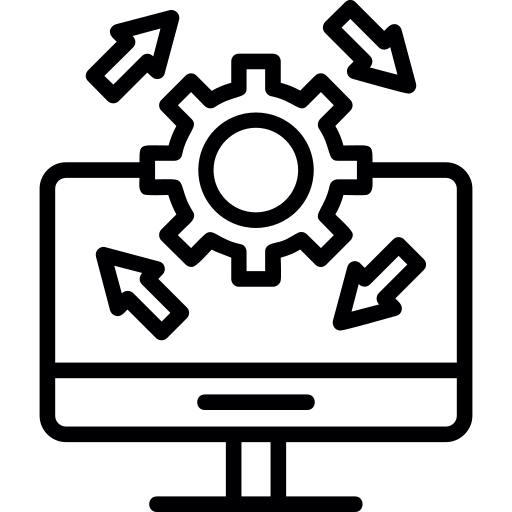
Continuously Improve Performance by Further Training the Model
The performance can be improved by further training the model using the data collected during operation. It is also possible to improve the false positive rate with a little manual work by allowing an inspector to specify the types of defects to be excluded.
Use Case – Optical Sheet Production Process
Due to the nature of optical sheets using light scattering, it is not easy to accurately detect defects in intermediate layers or surfaces. Traditionally, people used to visually determine defects based on background lighting. As a result, there is a problem that the defect detection rate is not constant depending on the proficiency of the inspector. That’s why we need a machine vision solution to accurately and automatically detect and classify all microfaults for many production models.
Normal image data is collected using a number of 4K Mono Line Scan Cameras for each reflected and transmitted light. We first train a fault detection deep learning model based on Anomaly Detection using this image dataset, and then further implement a classification model for the fault type.
Accurate defect detection using this solution reduced the defect rate of the final shipment by 35%. In addition, consistent quality management is possible regardless of the proficiency of the inspector.