DeepFHM
Deep learning-based Facility Health Management
Deep learning-based Facility Health Management (DeepFHM) is a solution to support maintenance strategies based on advanced Prognostics and Health Management (PHM) technology.
DeepFHM collects time-series data of operating conditions and states through vibration, acoustic, and current sensors, and monitors the real-time status of facility or products to detect faults from the initial stage of occurrence. For detected faults, diagnostic results including location, type, and severity are presented. Based on the results of this diagnosis, it provides predictive maintenance guidance information for health management.
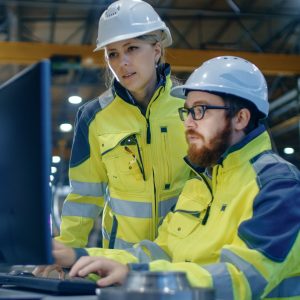
Possible to Detect Faults for the Entire Facility to Be Monitored
It is possible to detect faults for the entire facility to be monitored. This requires the installation of a significant amount of sensors depending on the size of the facility and the construction of large-scale models for data processing and analysis. In general, it is more efficient to perform predictive maintenance by limiting to specific components with high failure frequency or high criticality. The followings are typical components to be monitored: motors, gears, bearings, pumps, filters, compressors, cooling fans, pipes, valves, electrical devices, heat exchangers, presses.
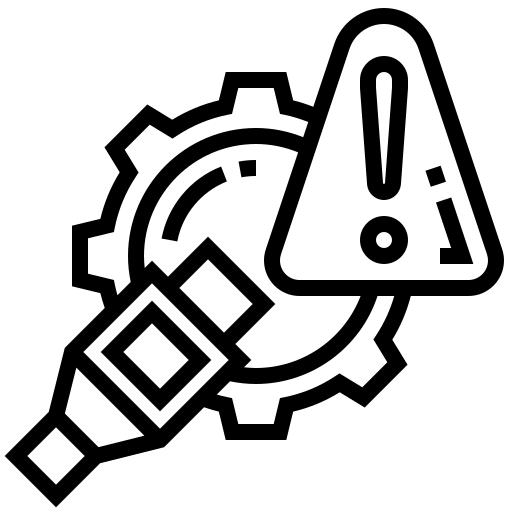
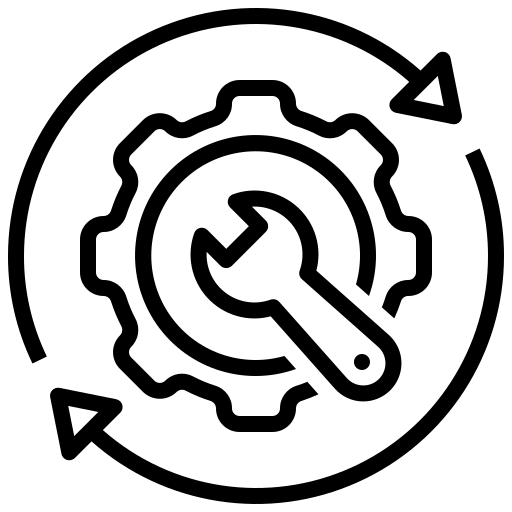
Efficient Predictive Maintenance
All factories need to minimize downtime and waste of resources due to unplanned facility faults. Reactive maintenance or preventive maintenance alone cannot avoid the problems of lower facility utilization, higher defect rates, and higher repair costs. In general, predictive maintenance is known to have a cost savings of 12% compared to preventive maintenance and 40% compared to reactive maintenance. For efficient predictive maintenance, it is necessary to immediately detect the occurrence of a fault through real-time monitoring of the facility status. In addition, when a fault occurs, it is also necessary to understand its location, direct cause, and degree.Prediction of the future condition or remaining useful life (RUL) of equipment can be used as important information for predictive maintenance.
Increase Profits
Real-time fault response is possible by utilizing accurate facility fault diagnosis information, and timely predictive maintenance is possible through accurate facility fault prognosis. Efficient predictive maintenance can reduce costs, minimize losses, improve quality, and finally increase profits.
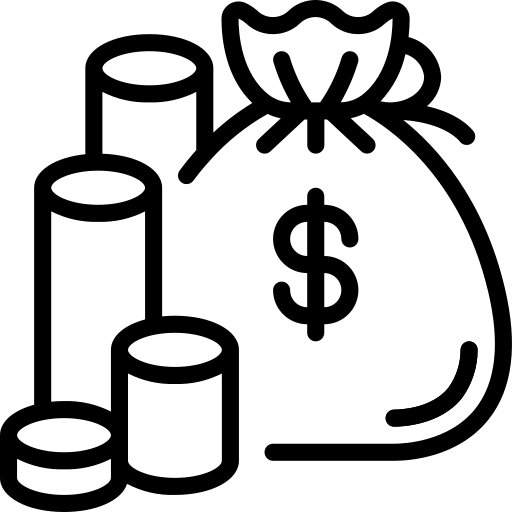
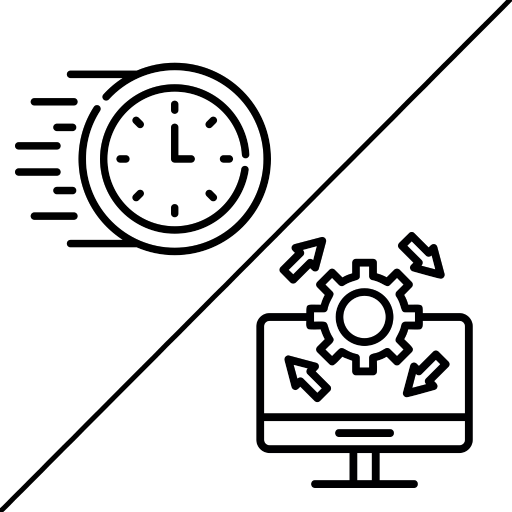
Fast Initial Installation, Continuously Improve Performance by Further Training the Model
DeepFHM enables rapid initial installation of fault detection functions with minimal data collection. In addition, it is possible to further train the model for performance improvement using the data collected during operation.
Use Case – Steam Generation Process
The operation efficiency of the entire process may be reduced due to a sudden failure of the boiler facility for steam generation. A system is required to detect and diagnose all potential failure areas where performance degradation may occur.
Temperature, pressure, flow and vibration sensors can be installed in the potential fault area to build a normal set of time series data for the entire facility and detect faults with the Anomaly Detection deep learning model. The machine learning model, which classifies the location and cause of the failure, enables rapid response to the failure situation.
The solution reduces downtime due to failures by 41% and improves overall process utilization by minimizing outages.