AI R&D
Detection of Harmful Gases
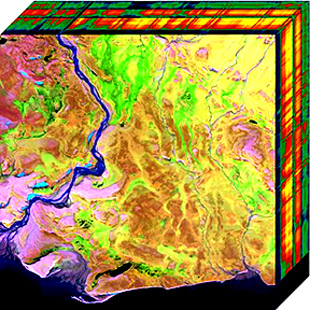
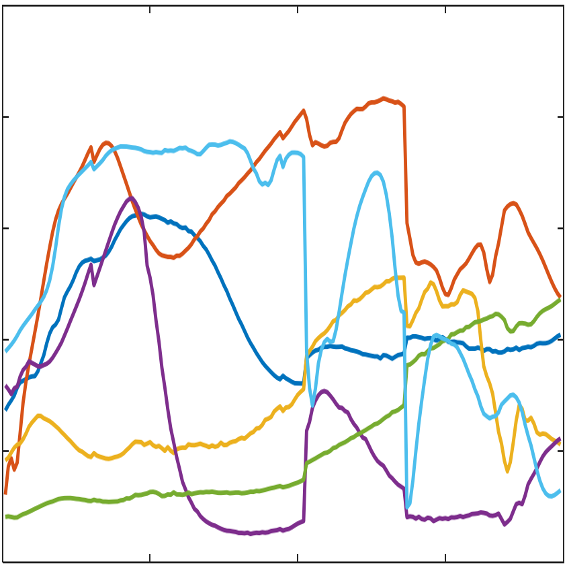
Recently, the spread of fine dust and the increase in carbon dioxide emissions are emerging as major social problems. Since fine dust is usually formed by a causal gas that acts as a precursor, it is an issue related to the detection of harmful gases that can be addressed in the same context as carbon dioxide. Although the source of these harmful gases is managed through regulation, the absence of management due to unauthorized emission is also reaching a serious level. The starting point of the problem is that most harmful gases are basically difficult to identify with human eyes. The technology that can detect the presence of harmful gases in the air is Hyper-Spectral Imaging (HSI) technology. HSI composes 2D image information in the form of hyperspectral cubes in the spectrum band of electromagnetic waves. By analyzing this hyperspectral cube, the composition, state, and characteristics of the object can be derived.
It is a common phenomenon that spectral mixture occurs as the sum of individual spectra from different materials, rather than the spectra of only one material appearing in one pixel of the captured hyperspectral image. In this case, if we try to detect only one substance using the endmember’s unique spectral properties, it will be distorted by the spectrum of other substances and we will not be able to accurately detect it. This R&D is to find individual substances by using the Spectral Unmixing technique, which analyzes the composition ratio of individual substances in each pixel where the spectra of multiple substances are combined. Individual substances, that is, end members, are selected from the main sources of fine dust, carbon dioxide and carbon monoxide. This R&D aims to improve performance by applying a deep learning method that shows excellent performance in image analysis for spectral mixing analysis. In order to find the ratio (abundance) of the gases, a network that combines an autoencoder and CNN, which is one of unsupervised learning, is used. It is a structure in which the latent code of the autoencoder network becomes a vector representing the ratio of substances to be obtained.
Road Crack Detection
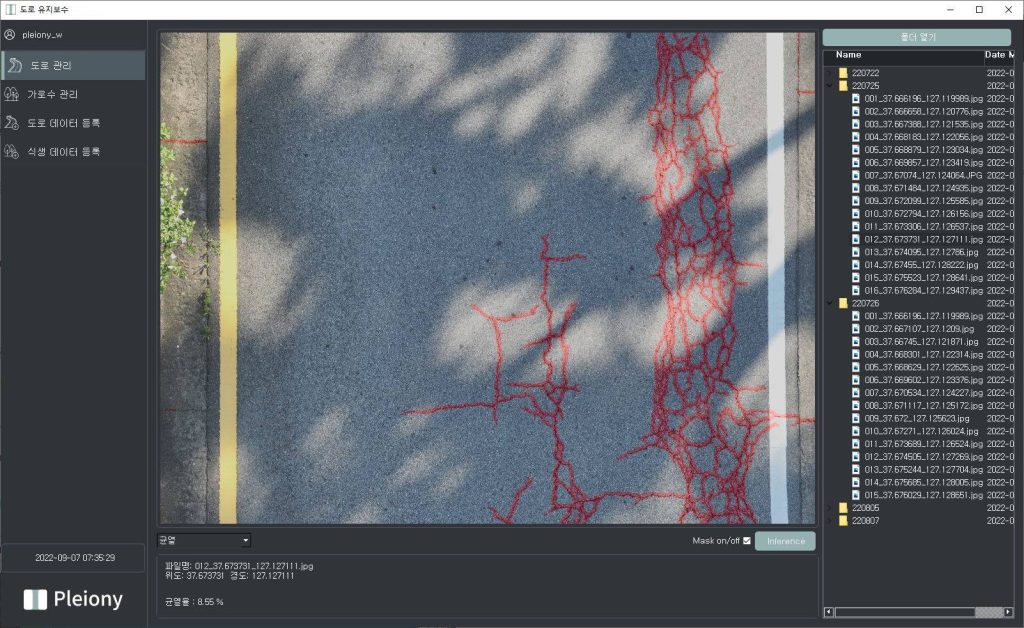
Road maintenance is becoming more important not only in developed countries but also in Korea, and the importance of maintenance is emerging as the number of aging road facilities increases. The size of domestic roads has increased 2.1 times over the past 30 years, and the number of maintenance targets reached 107,527 km as of 2016. The number of major road facilities is increasing due to the continuous new road construction, and the deterioration of road facilities is increasing year by year. Domestic road maintenance costs rose from KRW 934.3 billion in 1995 to KRW 2.30 trillion in 2014, and it is expected that the scale of road maintenance costs will continue to rise in the future. Therefore, there is a need for a new method to increase the efficiency of maintenance, and the need to establish an optimal road maintenance management system through maintenance at an appropriate time is emerging.
Extracting accurate indicators of road conditions is critical to optimizing road maintenance schemes. Various subjective and objective indicators have been defined, and many attempts have recently been made to automate the calculation of these indicators. In general, maintenance work is performed when the crack rate is greater than 20%, and it is important to accurately calculate the crack rate for the crack area including the pothole. Using a recent segment detection deep learning model, it has become possible to accurately detect and identify cracks and pothole areas from drone-photographed road pavement images. The area values for the segmented area are used for the following crack rate calculation, allowing an accurate understanding of road conditions.
This crack rate value will be used in the calculation of Present Serviceability Index (PSI), National Highway Pavement Condition Index (NHPCI), Maintenance Control Index (MCI), Seoul Pavement Index (SPI), and Highway Pavement Condition Index (HPCI).
Image Watermarking
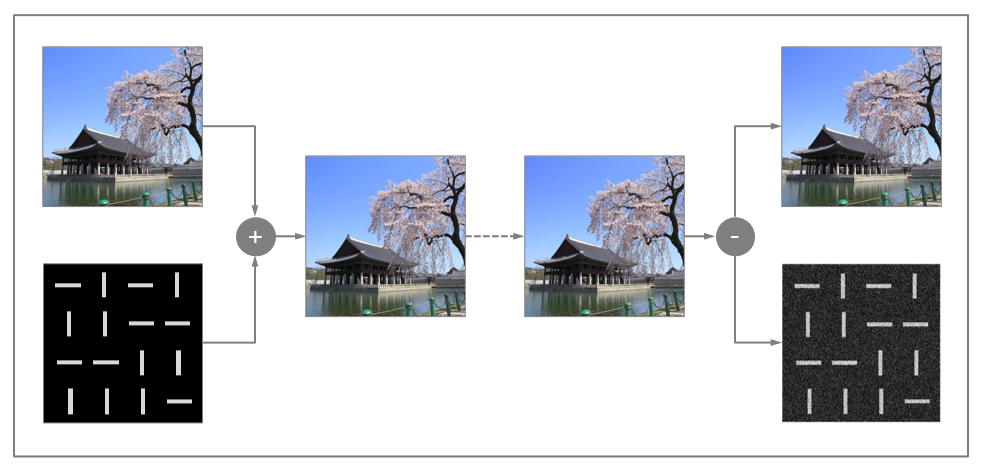
Image watermarking refers to a technique for covertly inserting and extracting information into an original digital image. It can be used to create and transmit the watermarked image by hiding information in the original image so that it is not visually revealed. This is a method that allows the receiving party to check the watermark information only if it has permission. This watermarking technology can be utilized for many applications. For example, it is possible for the copyright information of an image to be hidden in the form of a watermark. Meta information such as object type and bounding box may be hidden without visible changes to the original image. It can also be used for the purpose of judging fake images or manipulated images posted on social media sites. It hides invisible marks all over the image to detect even the slightest change.
For image watermarking to work well, it is important that the quality of the watermarked image is maintained. It is for the original use of the image, and for the purpose of making it visually obscure that the watermark is inserted. The presence of a watermark should not be detected by computer analysis. Also, even if the watermark image is degraded or damaged, the watermark information must survive. This robustness of watermarking is very difficult to realize considering trade-off relationships due to its conflicting characteristics with the concealment of watermarks. Watermark extraction must be done without any information about the original image, and this blindness nature makes watermarking technology more difficult to implement. Image watermarking technology is a field that has been continuously researched for about 30 years, but it can still perform basic functions only under limited conditions and has many vulnerabilities to various types of attacks.
In order to overcome these problems, research to apply deep learning technology to image watermarking has recently attracted a lot of attention. The key reason is that deep learning models show excellent ability to approximate and generalize complex features of images through representation learning. The advantage of applying deep learning technology is that it can automatically learn multiple levels of features for image watermarking using training data. By using these features, it is possible to make the watermark well hidden and to survive multiple attacks. By applying image watermarking technology to each frame of video, it can also be used for video watermarking. Due to the nature of the video, which is compressed based on the relationship between consecutive frames, inserting a watermark in every frame may decrease the extraction performance. However, not all information in a single frame remains in the compressed frame, which requires a different kind of robustness technique than in the case of images.